Table of Contents
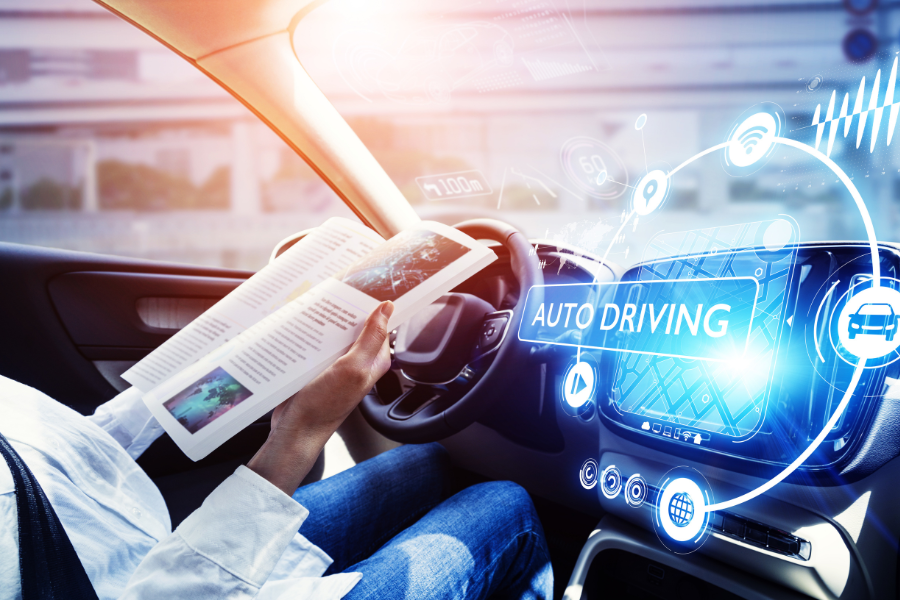
The advent of autonomous vehicles is a revolutionary milestone in the history of transportation. At the core of this innovation lies the intricate and advanced fields of Artificial Intelligence (AI) and Machine Learning (ML). These technologies are not just add-ons but are fundamental to the operation of driverless cars. In this comprehensive exploration, we delve into how AI and ML are being utilized in autonomous driving, focusing on three crucial aspects: Data Processing, Machine Learning Algorithms, and Deep Learning.
Data Processing in Autonomous Vehicles
Understanding the Data Deluge
Autonomous vehicles are equipped with an array of sensors, including cameras, Lidar (Light Detection and Ranging), radar, GPS, and others, each continuously generating vast amounts of data. This data is complex and multidimensional, encompassing everything from the vehicle’s surroundings to its internal state.
Real-Time Processing and Decision Making
The primary challenge is processing this data in real-time. AI systems in autonomous vehicles must quickly interpret and react to a continuously changing environment. This involves identifying objects, assessing their speed and trajectory, and understanding road conditions, all while navigating and adhering to traffic rules.
Data Fusion for Enhanced Perception
Data fusion is a critical aspect of this process. It involves combining data from different sensors to create a comprehensive understanding of the vehicle’s environment. For example, while cameras provide detailed visual information, Lidar offers precise distance measurements. AI algorithms integrate these inputs to develop a 360-degree view of the surroundings, crucial for safe navigation.
Machine Learning Algorithms in Autonomous Driving
Learning from Experience
A defining feature of ML is its ability to learn from data and improve over time. In the context of autonomous vehicles, this means learning from past driving experiences and continuously enhancing performance.
Algorithm Diversity
Various ML algorithms play different roles, such as decision trees for rule-based scenarios, and reinforcement learning for adapting to new situations. Each algorithm has its strengths, and their combination leads to a robust and adaptive driving system.
Continuous Improvement
The beauty of ML in autonomous driving is its continuous improvement cycle. As vehicles encounter diverse scenarios, the algorithms learn and evolve, leading to progressively safer and more efficient driving strategies.
Deep Learning and Sensor Data Interpretation
Neural Networks at the Core
Deep Learning, a subset of ML, is particularly relevant in interpreting sensor data. Neural networks, which are inspired by the human brain, excel at recognizing patterns in large datasets.
Interpreting the World Through Sensors
Deep neural networks are adept at making sense of the complex data from various sensors. They can differentiate between pedestrians, other vehicles, and static obstacles, even under varying weather and lighting conditions.
Advanced Decision-Making
These networks do not just interpret data; they also contribute to decision-making. By analyzing patterns in the sensor data, they predict the actions of other road users and decide the safest course of action for the vehicle.
The Future of Neural Networks
As deep learning algorithms become more sophisticated, we can expect even more nuanced understanding and decision-making from autonomous vehicles. This could lead to vehicles that can navigate more complex environments and handle more challenging driving scenarios.
Conclusion: The Road Ahead
The integration of AI and Machine Learning in autonomous vehicles is not just about replacing the human driver. It’s about creating a new paradigm in transportation that is safer, more efficient, and more accessible. As we continue to refine these technologies, the dream of fully autonomous driving becomes increasingly a reality. The journey of AI and ML in this field is just beginning, and its potential is as vast as the open road.